Synthetic Data and Human-in-the-Loop: Balancing Automation with Human Expertise
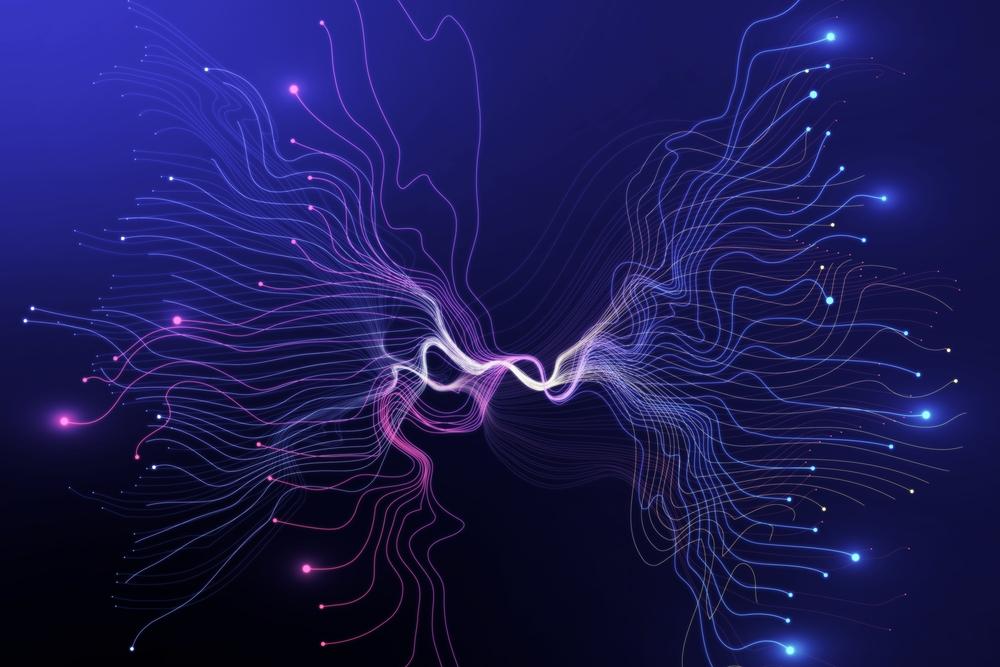
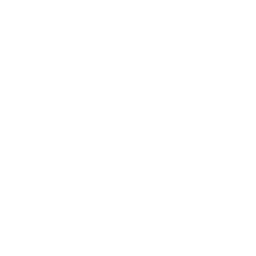
The world of artificial intelligence (AI) and machine learning (ML) has evolved at an unprecedented pace over the last few years. With the advent of new technologies, algorithms, and tools, businesses across industries are looking to automate tasks, make data-driven decisions, and improve efficiency. However, the success of AI and ML heavily depends on data quality, quantity, and diversity. This is where synthetic data and the concept of human-in-the-loop come into play.
The Role of Human-in-the-Loop in Artificial Intelligence and Machine Learning
Human-in-the-loop refers to the involvement of human experts in the AI and ML process. The idea is that humans can provide context, feedback, and guidance to algorithms, which can ultimately improve the accuracy and relevance of the outputs. The role of humans in the loop is especially important when it comes to data labeling, training, and validation. While machines can process vast amounts of data at high speeds, they often struggle with ambiguity, complexity, and nuance. Humans can help address these issues by providing accurate and consistent annotations, identifying edge cases, and preventing bias.
What Is Synthetic Data and How Does It Work?
Synthetic data refers to artificially generated data that mimics real-world data. It can be created using algorithms, simulations, or a combination of both. Synthetic data can be used to supplement or replace real-world data, especially when the latter is scarce, biased, or sensitive. Synthetic data can also help improve data diversity, generate new insights, and reduce the cost and time required for data collection.
How to Approach Synthetic Data for Human-in-the-Loop
The rise of synthetic data has significant implications for human-in-the-loop. On the one hand, synthetic data can reduce the need for human-generated data, which can be time-consuming, expensive, and error-prone. On the other hand, synthetic data can also introduce new challenges for human-in-the-loop. For example, humans may need to validate the quality and relevance of synthetic data, ensure that it reflects real-world scenarios, and detect and address any biases or errors in the generation process.
So, how should we approach synthetic data and human-in-the-loop? Here are some key considerations:
- Determine the use case: Before deciding to use synthetic data, it is essential to identify the specific use case and the problem it is trying to solve. Synthetic data is not a silver bullet and may not be appropriate for all situations. It is crucial to consider factors such as data availability, data quality, data diversity, and data privacy.
- Validate the quality of synthetic data: Synthetic data should be validated to ensure that it is of sufficient quality and relevance for the intended use case. Validation can be done by comparing the synthetic data to real-world data, conducting statistical analysis, and testing the performance of AI and ML models trained on synthetic data.
- Keep human experts in the loop: Even with synthetic data, human experts should be involved in the AI and ML process. Humans can provide feedback, validation, and oversight, which can help improve the accuracy and relevance of the outputs. Human-in-the-loop can also help identify biases and errors in the generation and use of synthetic data.
- Be transparent and ethical: As with any data, it is essential to be transparent and ethical when generating, using, and sharing synthetic data. Synthetic data should not be used to misrepresent or manipulate real-world data. It is also essential to consider the privacy implications of synthetic data, especially if it contains personal or sensitive information.
Navigating the Complexities of Synthetic Data and Human-in-the-Loop
Synthetic data and human-in-the-loop are two important concepts that can help improve the accuracy, efficiency, and relevance of AI and machine learning. While synthetic data can reduce the need for human-generated data, it can also introduce new challenges for human-in-the-loop. It is essential to approach synthetic data with caution and involve human experts in the process. Transparency and ethical considerations should also be a priority.
DataForce specializes in providing human-in-the-loop and data services that help businesses leverage the power of AI and ML while ensuring data quality, accuracy, and ethical use. Our experienced team of data scientists and experts can help you identify the right use case for synthetic data, validate its quality, and provide oversight and guidance throughout the AI and machine learning process.
To learn how DataForce's human-in-the-loop and data services can enhance your AI and machine learning projects with quality, accuracy, and ethical use, visit our website or contact us today.
By Brad Hastedt
Director, AI