The Power of Data Collection for Mitigating Bias in Generative AI
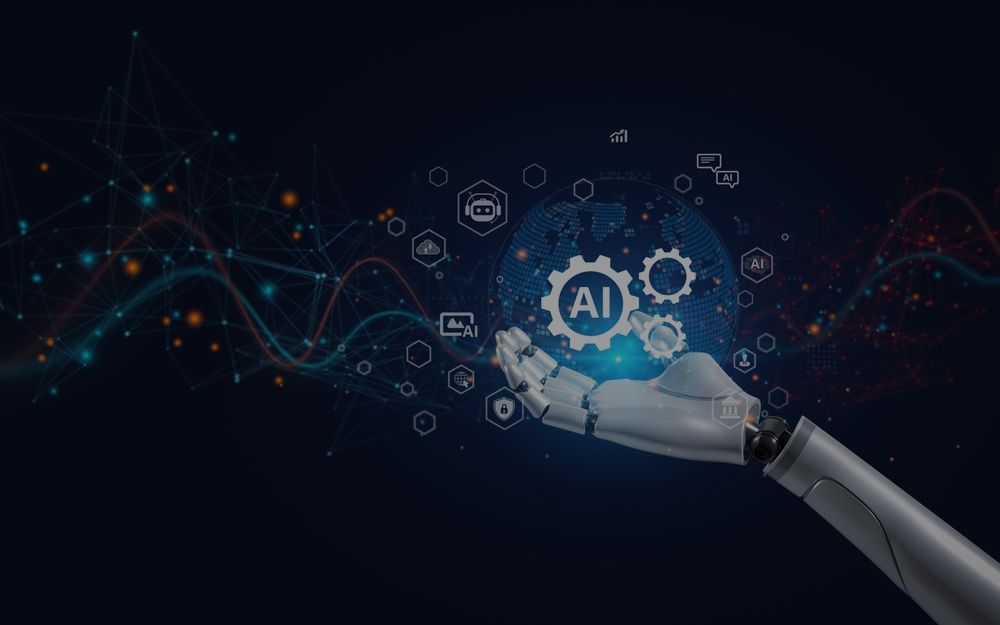
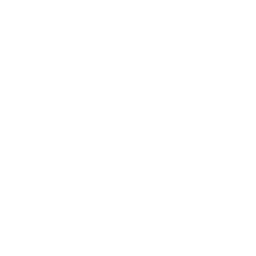
When it comes to generative AI (GenAI), the role and value of data cannot be overlooked. Data powers these models, and the quality of training data significantly impacts the systems’ performance, accuracy, and overall quality. As the saying goes: “Junk in, junk out.” This is especially true in the field of GenAI, where models are trained to create new content.
The Importance of High-Quality Data Collection
The quality of an AI model’s output depends on the quality of the data used to train it. This is even more true for GenAI models, where the output is new content that mimics its training datasets. So, it’s crucial to try to eliminate even unintentional biases in a GenAI model, such as cultural, gender, racial, or socioeconomic biases. Data that lacks diversity or is inaccurate may perpetuate human stereotypes or reinforce these existing disparities through the model’s output. However, a model trained on diverse and factually sound data will mitigate the appearance of biases and hallucinations. This process requires care and precision.
Even in seemingly robust datasets characterized by diversity, accuracy, and high quality, biases may still unintentionally creep in, leading to skewed results, especially in cases where the content is not carefully curated. For instance, consider models trained on historical workplace data tasked with generating images and resumes for various professions. Although the dataset might encompass both younger and older professionals, it may overrepresent male professionals among the older age group, inadvertently reinforcing gender bias in the workplace. Similarly, if a model isn't equipped to recognize harmful or offensive language, it might replicate such undesirable behaviors.
To effectively reduce the likelihood of unwanted and skewed outputs and promote the ethical and inclusive use of generative AI systems, it is imperative to conduct a comprehensive data collection.
How to Train Your Generative AI Model
To mitigate bias and ensure the accuracy of GenAI models, it is important to adapt rigorous data collection and model review practices and techniques:
- Diverse Representation: Include samples and datasets from different demographics, cultures, and backgrounds. This ensures diversity and inclusivity in the collection, which broadens the model’s understanding and promotes equitable outcomes.
- Bias Detection and Mitigation: Set up mechanisms to detect and mitigate biases in the dataset, whether it involves manual inspection, algorithmic bias detection tools, or crowdsourcing validation.
- Continuous Improvement: Datasets should be continuously refined and updated depending on feedback, emerging patterns, and changing ethical norms to ensure the dataset’s relevance and integration over time.
Ensuring Bias Mitigation in Generative AI Models
By prioritizing diversity, inclusivity, and ethical considerations throughout the model training process, GenAI developers can maintain ethical standards.
At DataForce, we equip our customers with data solutions that can be used to train and improve generative AI models to be more accurate and unbiased. Learn more about our generative AI training datasets and data collection services, or contact us today to start fine-tuning your model.
By Ashley Smith, Account Executive, AI