Improving Search Relevance: High-Quality Data with a Personal Touch
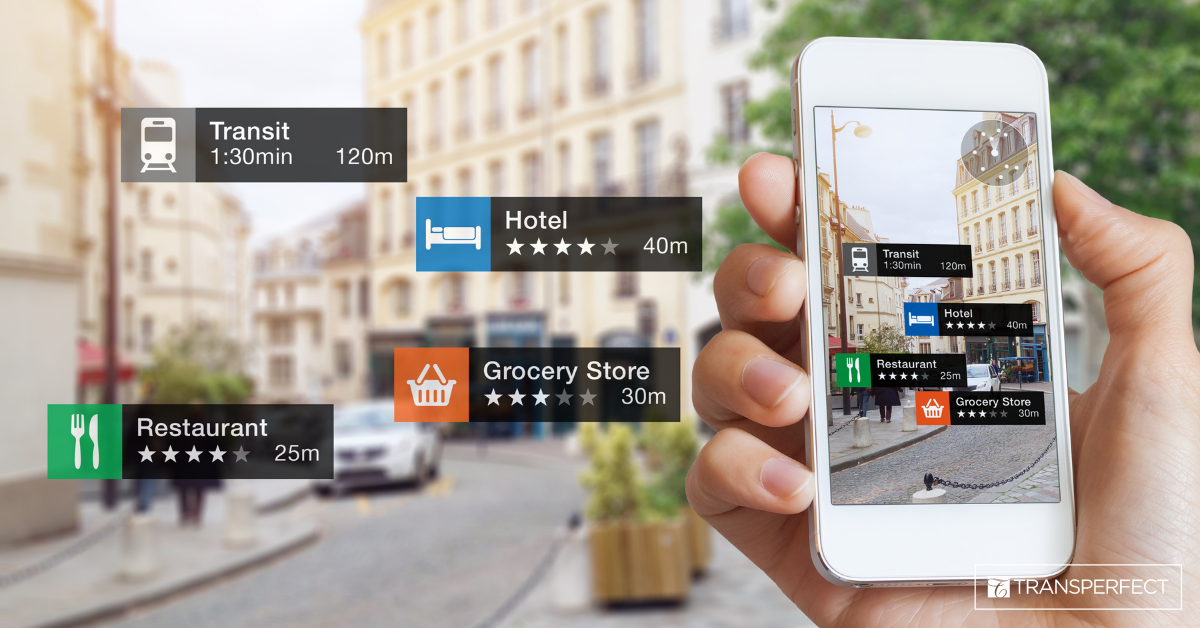
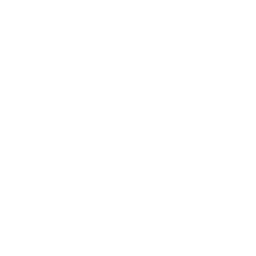
Have you heard of the human-in-the-loop (HITL) model?
In a nutshell, it’s a method that’s put in place to achieve what humans and machines can’t do without the other. It helps provide high-quality data with a personal, human touch.
Many organizations have deployed the HITL model to help advance their products, features, and search algorithms. It collects inputs and measurements from humans to measure, train, and optimize the relevance and quality of results for end-users to create a better user experience.
So, what does this have to do with improving search relevance?
What Is Search Relevance?
Search relevance is a measurement of how relevant results, or sets of results, are to a user who is looking for information on the internet, products on commercial or retail websites, or specific content within an enterprise.
These measurements help organizations understand how relevant, meaningful, and, most importantly, useful search results are to their users. These learnings support the development, improvement, and fine-tuning of their search algorithms. Helping your users discover and find the most relevant information or the right product easily and conveniently leads to a better user experience and user retention.
Improving and training search algorithms relies on many relevance signals. It consumes vast amounts of training data, which, in turn, requires a large number of human evaluators to create sufficiently large training data sets. This becomes even more so when products are global and support different languages and markets.
Human evaluators label, annotate, and rate high volumes of data to generate meaningful insights and relevance signals. Doing so supports improvements in search algorithm relevance standards and quality.
Typically, this type of work is managed on secure platforms. Using a vetted community of human evaluators, such as TransPerfect DataForce, directly connects training data workflows to the community.
Quality and Accuracy
There is nothing worse for search algorithms or machine learning models than bad training data or biases.
That’s where human evaluators come in to play.
Human Evaluators
Human evaluators are subject-matter experts who are pre-screened, qualified, and working within strict guidelines to ensure high-quality training data. They ensure personal opinion and biases do not influence the data.
In search relevance, training data can consist of simple query/result pairs. Human evaluators assess the relevance of the query against a single or a more holistic evaluation. The entire result sets are then evaluated either on their own or side by side.
Evaluators must research and understand the user intent in order to determine how relevant the result or result set was to the user query and to what level it satisfies the user’s intent.
Many factors can influence the quality of the training data and understanding user intent is critical in this process.
Queries can be ambiguous. They can have broad intents and different meanings in different countries, languages, or domains. To deal with these factors, human evaluators need to have expert judgment, critical thinking, cultural awareness, and, most importantly, intimate local knowledge of their market or domain.
The Evaluation Process
To measure quality improvements and support launch decisions, a robust evaluation process should be in place. This will inform launch decisions that a new release will actually improve relevance standards and quality, and thereby the user experience.
A common way of achieving this is by creating measurement sets that are used to measure the relevance and quality of a new release against a baseline measurement taken on the current release. Human evaluators again play a part in this as they evaluate the results or result sets, enabling the calculation of a relevance and quality score against that baseline.
Search relevance plays a vital role in delivering quality results that meet the expectations and needs of your users. HITL models bring the human factor into these measurements, ensuring that user intents are understood, ambiguity is sorted through, and data sets are accurate and relevant.
By having humans help sort through all this data, continuously iterating on your search relevance measurements and embedding them into the development cycle of new releases allows organizations to make better and smarter decisions.
To learn more about search relevance or to explore how DataForce can help you achieve your goals, take a look at our case studies or get in touch.
By Gabriel Vollmeyer,
Director of Strategic Accounts,
DataForce by TransPerfect