Enhancing User Experience and Product Development Through Data Annotation and Large Language Models
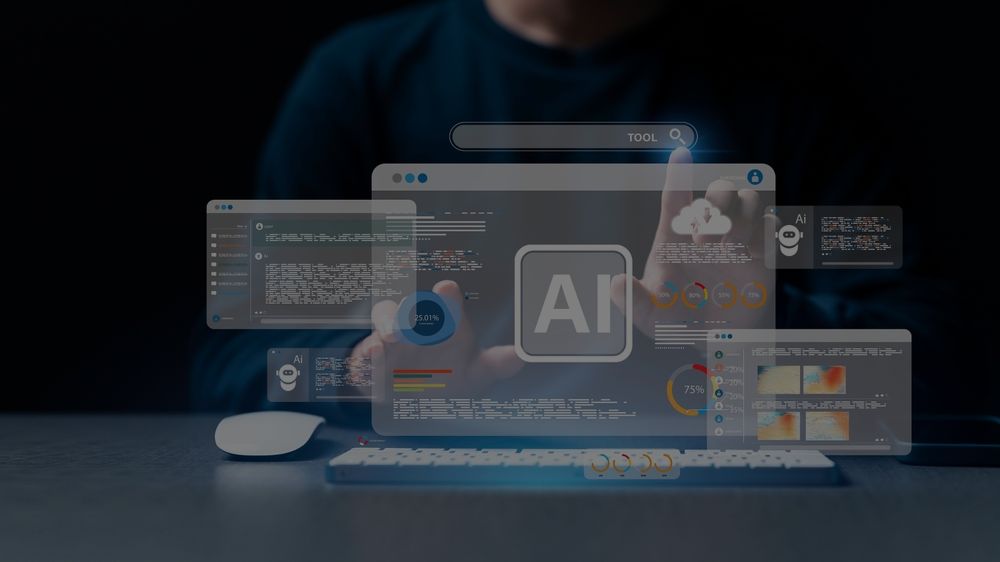
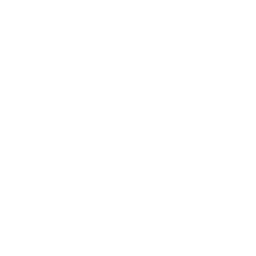
The success of AI-powered products heavily relies on rigorous testing and continuous improvement. While advancements in machine learning have propelled innovation, human oversight remains crucial. Data annotation along with the utilization of large language models (LLMs) play a pivotal role in refining user experience and product development.
Leveraging Data Annotation for User Experience and Product Development
Data annotation is the foundation of high-quality training data, which is essential for machine learning models to operate efficiently and ethically. Annotators thoroughly analyze and label data to mitigate model bias and, in turn, enhance the user experience. Additionally, annotated data provides invaluable insights that allow companies to refine their products in response to real-world usage patterns and customer feedback. The continuous refinement of machine learning models ultimately enables more refined, user-centric products.
How LLMs are Utilized in Data Relevance Rating and User Experience Testing
Large language models are essential in various aspects of data relevance rating and user experience testing, allowing businesses to fine-tune their products to better meet user expectations.
Performance Assessment
LLMs enable accurate evaluation of many performance metrics such as fluency, accuracy, coherence, and subject relevance. These models provide a standardized framework where developers can test the performance of their models in understanding and generating relevant content. Additionally, developers can find areas of improvement and obtain significant insights into model performance by thoroughly comparing LLM-generated outputs to annotated data.
Model Comparison
LLMs facilitate the comparison of models, simplifying the decision-making process for developers. Through rigorous testing and evaluation, developers can determine which model best meets the criteria for both user experience and data relevance. Businesses can implement the most effective and efficient solutions through comparative analysis using LLMs, ensuring outstanding performance and user satisfaction.
Bias Detection and Mitigation
LLMs can help detect and correct biases in machine learning models and datasets. Through comprehensive analysis, they can identify bias tendencies in data and model outputs, allowing developers to address these concerns ahead of time. LLMs for bias detection can help companies create fair and inclusive user experiences, increasing trust and credibility in their products.
User Satisfaction
LLMs evaluate criteria related to user experience such as coherence, relevance, and diversity, offering insightful feedback on the user experience. These models analyze user-generated content and interactions to determine the overall quality and usefulness of AI-powered products. Personalized experiences, content recommendations, and user interfaces are continuously improved with the use of insights obtained from these models. In the end, this results in increased user retention and satisfaction.
Unlock the potential of LLMs and Data Annotation
The integration of large language models and data annotation for user experience testing and data relevance rating maximizes the efficiency, relevance, and diversity of data, driving innovation in user experience and product development. Adopting these approaches allows businesses to continuously improve their AI-powered products and consistently provide the best performance and user satisfaction.
Discover the power of data-driven insights and build an unmatched user experience through DataForce’s personalized user studies services. Schedule a consultation with our experts today to discuss how DataForce can enhance your AI-powered products.
By DataForce