Bias Mitigation Services
Resolve unbalanced predictions and skewed decision-making with accurate machine learning model outputs
DataForce & Bias Mitigation
In an era where artificial intelligence increasingly influences decision-making, bias mitigation has become both a technical challenge and a moral imperative. As AI systems integrate further into everyday life, the risks of perpetuating social inequalities and functional inaccuracies grow. Bias in data can lead to incorrect predictions or inconsistent performance across different populations, particularly when training data lacks sufficient diversity. This weakens the reliability and adaptability of AI models, leading to flawed results in scenarios they weren’t properly exposed to.
Ethically, biased AI can perpetuate discrimination and unfairness, disproportionately affecting marginalized groups in critical areas like healthcare, law enforcement, and hiring. This only reinforces social inequalities and erodes trust in AI systems. To confront these issues, a holistic approach is necessary—one that includes technical solutions like algorithmic fairness and data diversification alongside human oversight and broader societal engagement. The future of AI hinges not only on intelligence but on justice, shaping technology that truly serves everyone.
Business Risks
Why it is important to mitigate bias:
- Reputational Damage
- Legal & Regulatory Risks
- Loss of Customer Trust
- Financial Losses
- Biased Decision-Making
- Operational Risks
- Loss of Innovation Opportunities
- Internal Cultural Issues
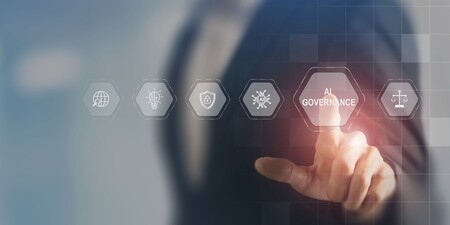
How It Works
Project definition and consultation:
Leverage our global consultants and SMEs across six continents to map out ethical considerations for your application and unearth potential gaps.


Data Collection:
Utilize our DataForce Contribute mobile application to collect data for video, audio, image, speech, and text to ensure datasets are balanced and reflect cultural and regional preferences.

Data Annotation:
Leverage 100% human judgments and technology to label at scale, allowing teams to test and iterate faster.

Data Management:
Leverage 100% human QA checks based on task type and expertise needed to spot inconsistencies and mitigate bias.
Bias Mitigation Services
- Localization
- Bias Detection and Mitigation
- Data Cleansing
- Red Teaming
- Content Moderation
- Prompt Writing & Re-writing
- Data Collection
- Data Annotation
Ethical AI
Ethical AI is essential for ensuring fairness, transparency, and responsibility in AI development and use. Without it, businesses face significant risks like reputational damage, legal issues, and the erosion of customer trust. Unethical AI can lead to biased decision-making, privacy violations, and regulatory fines, undermining operational efficiency and causing long-term harm.
Common Biases
An overfit model performs extremely well on training data but fails to make accurate predictions on real world data. This generally happens when models are too complex or niche and fail to understand the noise that will most likely come from real world data. Thus, the model has a hard time making generalizations across new data.
In many cases, there isn’t enough “new data” for AI models to train on. Some models, like LLMs, require massive amounts of training data, and data collection efforts can’t always keep up with the rapid societal, political, and economic changes we see month to month.
This occurs when AI systems are influenced by pre-existing beliefs. If a model builder unconsciously disregards data that doesn’t align with their perceptions, the model will likely be unable to find new patterns and instead reinforce the pre-existing beliefs.
This occurs when computer systems produce biased results that are discriminatory or unjust.
This occurs when the data used to train an AI system is not representative of the application's true intention. An example is building an AI model for car loan approvals but only collecting data for the top 1% of earners. The data should be just as diverse as the population, not a subset.
This is a form of bias where annotation groups unconsciously inject their own biases into the labeled data, resulting in various groups being discriminated against.
This occurs when certain groups of features are systematically excluded from the data used to train the AI model (e.g., an LLM that excludes certain languages).
This occurs when the context in which data was collected differs from the context in which the model is applied. An example of this would be a UK AI company developing and training a model for the US market with British English and terminology as the core of its training dataset. This might not translate very well to US lingo, terminology, and regional differences.
This is a common type of bias where individuals are grouped based on a few characteristics that lead to stereotyping (e.g., generalizing data based on age, race, income, location, etc.).
Success Stories.
We've partnered with thousands of companies around the world. View a selection of our customer stories below to learn more about how DataForce has helped clients excel in the global marketplace.
Thought Leadership.
DataForce Benefits.
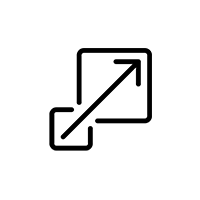
Scalable
Onboard 0-1000 annotators within weeks of project kickoff, leveraging a global community of over 130 million contributors
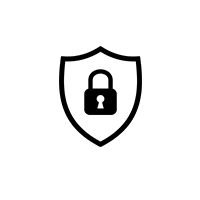
Secure
Your data is secure with SSAE 16 SOC 2, ISO 27001, HIPAA & GDPR
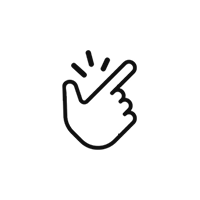
Seamless
Integrate directly with the DataForce annotation platform, yours or a third party of your preference
Let's work together!
Fill out the form and DataForce team member will respond shortly