Navigating the Bias Challenge: Medical Data for Ethical AI in Life Sciences
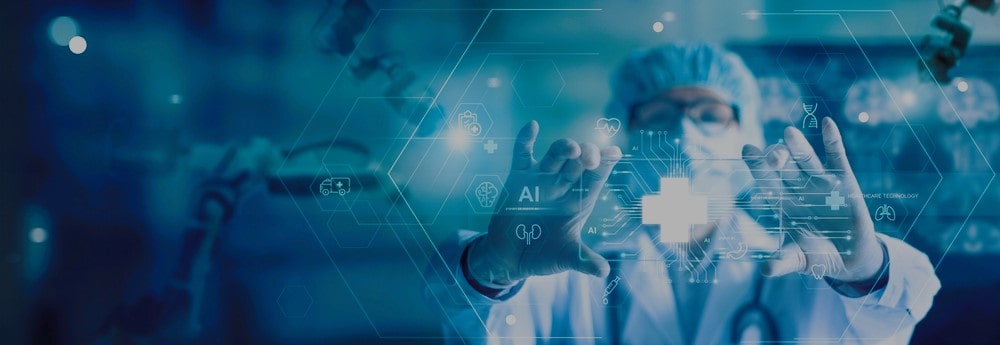
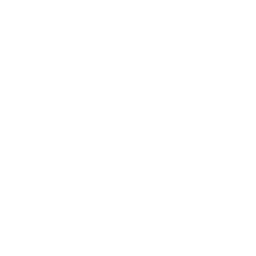
From drug discovery to personalized medicine, AI systems are broadly transforming how we approach health and disease. The clinical development life cycle generates enormous volumes of data, and its potential is fully realized through the strategic application of AI tools, such as data annotation for medical imaging and adverse event detection. However, the integration of AI into such a sensitive and critical field comes with an ethical imperative: ensuring the AI systems we deploy are free from bias.
The Bias Problem in Medical Data
Bias in clinical data often stems from historical health inequalities and disparities in healthcare access. For instance, if a dataset has an overrepresentation of a particular demographic, the AI trained on this data may not perform well for underrepresented groups. This can have serious clinical implications, including misdiagnosis or inappropriate treatment recommendations for those groups.
Another challenge is the bias introduced by the data collection process itself. Clinical data might be collected from healthcare facilities that cater to specific patient populations, which can inadvertently exclude other populations. In addition, certain medical conditions may be underdiagnosed in marginalized communities, leading to incomplete or skewed clinical data. Understanding these clinical biases in the data collection process is crucial to ensure that AI- driven systems are ethical and precise to provide equitable care to all patients.
Mitigating Bias: A Multifaceted Approach
1. Diverse Datasets
One fundamental step is to ensure the diversity of datasets.
- Pharmacovigilance and Patient Safety: Diverse datasets help safety teams keep track of and proactively address adverse drug reactions across diverse patient populations to ensure compliant reporting on the safety of medications.
- Social Listening/Monitoring: Gathering data across various patient demographics ensures a more comprehensive monitoring of patient sentiment, concerns, and feedback across different communities.
- Patents/IP Protection: Identifying potential patent infringements or intellectual property challenges during the development of medical devices is facilitated with diverse, insightful data.
- Diagnostics: Dynamic data in diagnostics aids the creation of more accurate and inclusive diagnostic tools that can help reduce the risks of misdiagnosis in underrepresented demographics.
2. Transparent Collection Practices
Transparency in data collection practices and clear documentation of where, how, and from whom data is collected are essential.
- Pharmacovigilance and Patient Safety: Transparent data collection practices improve accountability and traceability in pharmacovigilance efforts, maximizing the ability to promptly detect and document adverse events.
- Patents/IP Protection: Transparent collection processes can aid the protection of intellectual property by providing clear documentation of data sources and methodologies.
- Diagnostics: Transparent collection practices are crucial to validate the fairness and accuracy of diagnostic tools and gain regulatory approval.
3. Algorithmic Audits
Regular audits of datasets and algorithms are vital to detect and correct biases.
- Pharmacovigilance and Patient Safety: Pharmacovigilance audits can help effectively identify patterns or trends in adverse events, particularly when analyzing a variety of patient demographics.
- Social Listening/Monitoring: Audits ensure that algorithms used for social listening properly gather and analyze information from different communities and sources.
- Patents/IP Protection: Algorithmic audits examine data trends that may point to the improper use of protected information, which can assist in identifying any IP infringements.
- Diagnostics: Auditing diagnostic algorithms regularly preserves their ability to produce consistent and unbiased results for a variety of patient demographics, hence increasing diagnostic accuracy.
4. Inclusive AI Development
Involving stakeholders and including patients from diverse backgrounds in the AI development process can instill and incorporate valuable, dynamic insights into the needs and challenges of different groups.
- Pharmacovigilance and Patient Safety: Developing inclusive AI can help detect and route adverse events for reporting across a wider range of patient groups more accurately, improving patient safety.
- Social Listening/Monitoring: Involving diverse stakeholders in AI development guarantees that social listening tools consider the needs and concerns of various communities.
- Patents/IP Protection: Inclusivity in AI development contributes to ethical and legal considerations while protecting intellectual property in the medical industry.
- Diagnostics: Creating inclusive diagnostic training materials for AI technologies widens the scope to ensure patients with various medical problems and backgrounds can be served efficiently.
5. Continuous Education
Consistently educating and training AI developers and their users about the importance of ethical considerations and the potential consequences of bias is crucial for fostering a culture of responsible AI use in life sciences.
- Pharmacovigilance and Patient Safety: Continuous education highlights the importance of remaining informed on safety regulations, which is crucial in pharmacovigilance.
- Social Listening/Monitoring: Providing ethical AI training ensures that experts in social listening are aware of the possible consequences of biased data collection and processing.
- Patents/IP Protection: Education on IP protection is crucial for professionally navigating the intricate patent landscape in life sciences.
- Diagnostics: Ongoing education reinforces the importance of maintaining ethical AI standards in diagnostic tool development to avoid bias in final outputs.
6. Regulatory Compliance
Adhering to regulatory standards and guidelines for AI in healthcare ensures that ethical considerations are systematically addressed.
- Pharmacovigilance and Patient Safety: Adherence to regulatory standards is essential in pharmacovigilance to ensure patient safety and reporting compliance.
- Social Listening/Monitoring: Regulatory compliance enforces patient privacy in social listening efforts by adhering to data privacy and security requirements.
- Patents/IP Protection: Following IP regulations is critical for protecting patents and intellectual property in the health sciences industry.
- Diagnostics: Adherence to regulations maximizes the potential for safe and efficient applications of AI diagnostic tools in clinical settings and is a prerequisite for obtaining regulatory approval.
Envisioning an Ethical Future for AI in Life Sciences
Acknowledging and mitigating biases within AI applications is crucial to ensuring equitable health outcomes for all. As part of a broader industry push, there is an imperative to prioritize the creation of fair, transparent, and inclusive AI systems.
As we continue to harness AI's potential, safeguarding the principles of equity and trust that underpin the essence of healthcare is critical. At DataForce, our data collection and labeling services are designed to prioritize ethical considerations, ensuring your AI systems are trained on high-quality, diverse, and representative datasets.
Elevating real-world clinical data is seamless when you have thorough, precise, and efficient annotation resources in your AI repository. Discover how you can transform your clinical data with ethical, strategic AI by connecting with our team today.
By DataForce